2024-11-06
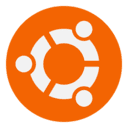
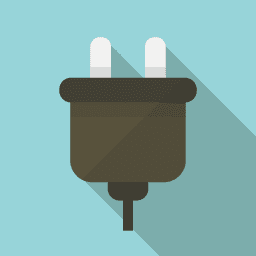
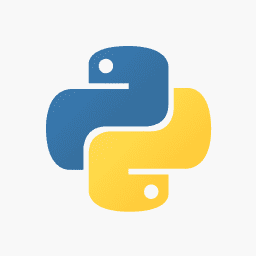
TSUKUMOのマルチGPUパソコンWA9J-X211/XTにUbuntu Server 20.04.2 LTSを導入する【1】
続きです。
1、CUDA Toolkitをインストール
どうやらこれにはNVIDIA GPUドライバ最新版も含まれているようで、前回のようにnvidia-470を入れてしまっていると、最新版とコンクリフトしてうまく入らない。
https://developer.nvidia.com/cuda-downloads
ここから、Linux→x86_64→Ubuntu→20.04→deb[network]を選択すると、スクリプトが表示されるので、その通り入力する。しかし、どのサイトもsudosudo連発馬鹿ですかと言いたい。最初にsudo -s してからコマンド打ったほうが簡単ではないか。
sudo -s cd # /rootで作業 wget https://developer.download.nvidia.com/compute/cuda/repos/ubuntu2004/x86_64/cuda-ubuntu2004.pin mv cuda-ubuntu2004.pin /etc/apt/preferences.d/cuda-repository-pin-600 apt-key adv --fetch-keys https://developer.download.nvidia.com/compute/cuda/repos/ubuntu2004/x86_64/7fa2af80.pub add-apt-repository "deb https://developer.download.nvidia.com/compute/cuda/repos/ubuntu2004/x86_64/ /" apt-get update apt-get -y install cuda
終わったらrebootする。
reboot
再起動以降爆音開始となるのは、正しくGPUを認識している証(GPUファン)ではあるが、うるさすぎるので、永続待機オプションをオフにして未使用状態では止める。
nvidia-smi -pm 0 Disabled persistence mode for GPU 00000000:17:00.0. Disabled persistence mode for GPU 00000000:65:00.0. All done.
永続待機状態にあるかどうかは、GPUを使っていないにもかかわらず、powertopコマンドでirq/91-nvidiaのようなプロセスが居て電力を食っていたら、永続待機であるので、上記オプションで切る。
環境変数のセットアップ
.bashrcなどに追記する。/usr/local/cuda-11.5が入ったはずであるので、
export CUDA_HOME=/usr/local/cuda-11.5 export PATH=$CUDA_HOME/bin:$PATH export LD_LIBRARY_PATH=$CUDA_HOME/lib64:$LD_LIBRARY_PATH
として読み込んだあと、
nvcc -V nvcc: NVIDIA (R) Cuda compiler driver Copyright (c) 2005-2021 NVIDIA Corporation Built on Mon_Sep_13_19:13:29_PDT_2021 Cuda compilation tools, release 11.5, V11.5.50 Build cuda_11.5.r11.5/compiler.30411180_0
コンパイラが読めればパスが通っている状態。
2、cuDNNライブラリのインストール
https://developer.nvidia.com/rdp/cudnn-download
から、入れたCUDAのバージョンと一致するものをダウンロードする。ユーザー登録が必要な場合JOIN NOWで登録。無料。
TSUKUMO-PCの場合、cuDNN Library for Linux (x86_64)→cudnn-11.5-linux-x64-v8.3.0.98.tgzをダウンロードする。
ダウンロードしたtgzファイルを展開する。
tar xvfzp cudnn-11.5-linux-x64-v8.3.0.98.tgz
suになって、念のため元のcuda-11.5ディレクトリのバックアップを取っておく。
suod -s cd /usr/local cp -Rp cuda-11.5 cuda-11.5.default
その後、展開したcudnnのcuda/include, lib64をcuda-11.5以下にコピーする。
cd cuda/include cp -Rp * /usr/local/cuda-11.5/include cd cuda/lib64 cp -Rp * /usr/local/cuda-11.5/lib64
環境変数のセットアップ
.bashrcなどに追記する。
export CUDNN_HOME=$CUDA_HOME
3、Python関係のインストール
Pythonは最新版が入っている模様。
python3 -V Python 3.9.7
pipが無いので、インストールする。
sudo apt install python3-pip
ここからは、suでシステム全体か、suせず自分専用で入れるか、好みが分かれるが、当方はsuで全体に入れたい派。
Pytorchのインストール
PytorchはTensorFlowと並ぶGPUをふんだんに使用する機械学習ライブラリである。
から、Stable→Linux→Pip→Python→CUDA 11.3を選ぶとコマンドが出るので、コピー&実行。
sudo -s pip3 install torch==1.10.0+cu113 torchvision==0.11.1+cu113 torchaudio==0.10.0+cu113 -f https://download.pytorch.org/whl/cu113/torch_stable.html ... Successfully installed numpy-1.21.4 pillow-8.4.0 torch-1.10.0+cu113 torchaudio-0.10.0+cu113 torchvision-0.11.1+cu113 typing-extensions-3.10.0.2
GPUの認識確認
python3 Python 3.9.7 (default, Sep 10 2021, 14:59:43) [GCC 11.2.0] on linux Type "help", "copyright", "credits" or "license" for more information. >>> import torch >>> print(torch.__version__) 1.10.0+cu113 >>> print(torch.cuda.is_available()) True >>> print(torch.cuda.device_count()) 2 >>> print(torch.cuda.current_device()) 0 >>> print(torch.cuda.get_device_name(0)) NVIDIA GeForce RTX 3090 >>> print(torch.cuda.get_device_name(1)) NVIDIA GeForce RTX 3090 >>> quit()
NVIDIA GeForce RTX 3090がPyTorchで無事認識できた。2枚のGPUを使ったPytorch使用コードは後日テストの上、アップしたい。
ここで、pythonインタープリタが起動中、import torch後、psやpowertopすると、
12.0 mW 112.6 s/s 3.0 Process [PID 2526] [irq/90-nvidia] 12.0 mW 111.8 s/s 3.0 Process [PID 2529] [irq/91-nvidia]
と、GPUのプロセスが居て、ファンが轟音であり稼働中であることがわかる。quit()としてPython, torchを終了すると、プロセスから居なくなり、ファンの轟音も消えるので、さきほどのnvidia-smi -pm 0の設定が効いているものと思われる。
TensorFlowのインストール
続けて定番TensorFlowを入れて、GPUの認識確認を行う。
旧ライブラリのアンインストール。最新版を入れるので念のため消しておく。
sudo -s pip3 uninstall -y tensorflow tensorflow-cpu tensorflow-gpu tf-models-official tensorflow_datasets tensorflow-hub keras-nightly keras
あらためて本体のインストール
sudo -s pip3 install -U tensorflow tf-models-official tf_slim tensorflow_datasets tensorflow-hub pip3 install git+https://github.com/tensorflow/docs pip3 install git+https://github.com/tensorflow/examples.git
エラーの対処
launchpadlib 1.10.13 requires testresources, which is not installed
と出ていたので、あらためてlaunchpadlib単体をインストール。
pip3 install launchpadlib Requirement already satisfied: launchpadlib in /usr/lib/python3/dist-packages (1.10.13) Requirement already satisfied: httplib2 in /usr/lib/python3/dist-packages (from launchpadlib) (0.18.1) Requirement already satisfied: keyring in /usr/lib/python3/dist-packages (from launchpadlib) (23.0.1) Requirement already satisfied: lazr.restfulclient>=0.9.19 in /usr/lib/python3/dist-packages (from launchpadlib) (0.14.2) Requirement already satisfied: lazr.uri in /usr/lib/python3/dist-packages (from launchpadlib) (1.0.5) Requirement already satisfied: setuptools in /usr/lib/python3/dist-packages (from launchpadlib) (52.0.0) Requirement already satisfied: six in /usr/local/lib/python3.9/dist-packages (from launchpadlib) (1.15.0) Collecting testresources Downloading testresources-2.0.1-py2.py3-none-any.whl (36 kB) Requirement already satisfied: wadllib in /usr/lib/python3/dist-packages (from launchpadlib) (1.3.5) Requirement already satisfied: SecretStorage>=3.2 in /usr/lib/python3/dist-packages (from keyring->launchpadlib) (3.3.1) Requirement already satisfied: jeepney>=0.4.2 in /usr/lib/python3/dist-packages (from keyring->launchpadlib) (0.7.1) Collecting pbr>=1.8 Downloading pbr-5.7.0-py2.py3-none-any.whl (112 kB) |????????????????????????????????| 112 kB 4.4 MB/s Installing collected packages: pbr, testresources Successfully installed pbr-5.7.0 testresources-2.0.1
また、kerasはtensorflow同梱のはずなのに何故かkeras単体もインストールされてしまっていて、import tensorflow.keras.などとやると、tensorflow.python.framework.errors_impl.AlreadyExistsError: Another metric with the same name already exists.という重複モジュールエラーが出てしまった。なので、kerasをアンインストールした。(ら動いた。)
pip3 uninstall keras
なお、keras_preprocessingはアンインストールしてはならない。おそらくこれはtensorflowがkerasを包含した際水面下で使用しているモジュールである。
バージョン確認
pip3 show tensorflow Name: tensorflow Version: 2.6.0 Summary: TensorFlow is an open source machine learning framework for everyone. Home-page: https://www.tensorflow.org/ Author: Google Inc. Author-email: packages@tensorflow.org License: Apache 2.0 Location: /usr/local/lib/python3.9/dist-packages Requires: grpcio, flatbuffers, tensorboard, protobuf, termcolor, gast, clang, numpy, wheel, opt-einsum, h5py, keras-preprocessing, wrapt, typing-extensions, absl-py, six, astunparse, tensorflow-estimator, keras, google-pasta Required-by: tf-models-official, tensorflow-text
GPUの認識確認
python3 Python 3.9.7 (default, Sep 10 2021, 14:59:43) [GCC 11.2.0] on linux Type "help", "copyright", "credits" or "license" for more information. >>> from tensorflow.python.client import device_lib >>> print(device_lib.list_local_devices())
出力結果
2021-11-06 20:11:53.599224: I tensorflow/core/platform/cpu_feature_guard.cc:142] This TensorFlow binary is optimized with oneAPI Deep Neural Network Library (oneDNN) to use the following CPU instructions in performance-critical operations: AVX2 AVX512F FMA To enable them in other operations, rebuild TensorFlow with the appropriate compiler flags. 2021-11-06 20:11:55.860076: I tensorflow/core/common_runtime/gpu/gpu_device.cc:1510] Created device /device:GPU:0 with 22311 MB memory: -> device: 0, name: NVIDIA GeForce RTX 3090, pci bus id: 0000:17:00.0, compute capability: 8.6 2021-11-06 20:11:55.860668: I tensorflow/core/common_runtime/gpu/gpu_device.cc:1510] Created device /device:GPU:1 with 22320 MB memory: -> device: 1, name: NVIDIA GeForce RTX 3090, pci bus id: 0000:65:00.0, compute capability: 8.6 [name: "/device:CPU:0" device_type: "CPU" memory_limit: 268435456 locality { } incarnation: 12562245482708196956 , name: "/device:GPU:0" device_type: "GPU" memory_limit: 23394975744 locality { bus_id: 1 links { } } incarnation: 12141721341066513620 physical_device_desc: "device: 0, name: NVIDIA GeForce RTX 3090, pci bus id: 0000:17:00.0, compute capability: 8.6" , name: "/device:GPU:1" device_type: "GPU" memory_limit: 23404806144 locality { bus_id: 1 links { } } incarnation: 8591648343889229398 physical_device_desc: "device: 1, name: NVIDIA GeForce RTX 3090, pci bus id: 0000:65:00.0, compute capability: 8.6" ]
無事認識できている。これまた、このコマンド後、psやpowertopすると、nvidiaプロセスが2個増えており、quit()すると、消えてファン音も無くなるので、永続使用設定オフが効いている。
Pytorchはこれからだが、TensorFlowについてはこのTSUKUMOマシンでデュアルGPUを使ったトレーニングを以下試して成功している。
【Windows】Python3.10+TensorFlow2.6-GPU+CUDA11.4+cuDNN8.2を動かす【4】
※本記事内容の無断転載を禁じます。
ご連絡は以下アドレスまでお願いします★
Debian 12でsshからshutdown -h nowしても電源が切れない場合
【Windows&Mac】アプリのフルスクリーンを解除する方法
Debian 12でtsコマンドが見つからないcommand not found
Debian 12でsyslogやauth.logが出力されない場合
Debian 12で固定IPアドレスを使う設定をする
Debian 12 bookwormでNVIDIA RTX4060Ti-OC16GBを動かす
【Debian】apt updateでCD-ROMがどうのこうの言われエラーになる場合
【Debian】あるはずのコマンドが無いと言われる場合
nohupで常時起動したPythonスクリプトがログを出力しない場合
Windows11+WSL2でUbuntuを使う【2】ブリッジ接続+固定IPの設定
【Windows10】リモートデスクトップ間のコピー&ペーストができなくなった場合の対処法
Windows版Google Driveが使用中と言われアンインストールできない場合
【Apache】サーバーに同時接続可能なクライアント数を調整する
【C/C++】小数点以下の切り捨て・切り上げ・四捨五入
【ひかり電話+VoIPアダプタ】LANしか通ってない環境でアナログ電話とFAXを使う
Intel Macbook2020にBootCampで入れたWindows11 Pro 23H2のBluetoothを復活させる
【OpenSSL1.1】Diffie-Hellman(ディフィ・ヘルマン)方式の鍵交換を行う
Googleファミリーリンクで子供の端末の現在地がエラーで取得できない場合